Machine Learning in Cybersecurity: Revolutionizing Cyber Protection
Machine Learning (ML) is a subset of Artificial Intelligence (AI) and involves the use of algorithms and statistical models to enable systems to improve their performance on a specific task over time, without being explicitly programmed. In cybersecurity, machine learning algorithms analyze patterns and learn from data to identify potential threats, making it a crucial tool in detecting and preventing cyber attacks.

Advancements in Threat Detection and Response
The dynamic nature of cyber threats necessitates a proactive and adaptive approach to security. ML excels in identifying new threats, including zero-day exploits and advanced persistent threats (APTs). By analyzing vast amounts of data through its algorithms it can detect anomalies that signify a potential security breach, often faster and more accurately than traditional methods.
Collaboration Between AI and Human Expertise
A balanced approach that combines the advanced algorithmic analysis with the nuanced understanding of human experts is crucial for an effective defense strategy. This collaborative model ensures a smooth integration of human decision-making in cybersecurity, fostering a more resilient and adaptable framework.
3 reasons why Machine Learning is critical against corporate cyber threats:
1. Integrating Emerging Technologies in Cybersecurity
The integration of emerging technologies like quantum computing and artificial intelligence is transforming cybersecurity. These advancements offer enhanced security capabilities but also present new challenges, requiring specialized skills for effective management and implementation. Their potential for bolstering cybersecurity measures is immense, necessitating an adaptive approach in security strategies.
2. Machine Learning in Identifying Insider Threats
Insider threats represent a distinct challenge in the field of digital security. Advanced algorithms excel in monitoring and analyzing user behaviors within an organization, to identify unusual patterns that may indicate a security risk from within. These sophisticated tools provide an essential layer of defense by continuously scanning for activities that deviate from the norm, thereby enhancing the organization's ability to address potential internal security breaches.
3. Enhancing Cyber Resilience through Advanced Analytics
In today's digital world, the importance of cyber resilience cannot be overstated. Advanced analytics play a crucial role in strengthening this resilience, providing insights that go beyond threat detection. By analyzing security data, organizations can gain a deeper understanding of attack patterns, which is invaluable in forming robust security policies and practices. This continuous analysis helps in adapting to the evolving nature of threats and maintaining a strong defense against potential attacks.
The Intersection of Cybersecurity and Regulatory Compliance
As technology evolves, so do the requirements for regulatory compliance in cybersecurity. Aligning cybersecurity practices with regulatory demands is not just about adherence to laws; it's about ensuring that organizations maintain the highest level of security while protecting sensitive data and customer information. This balance is essential for building trust and ensuring long-term success in the digital marketplace.
Cybersecurity with Continuous Learning Systems
The concept of continuous learning systems in cybersecurity is becoming increasingly important. These adaptive systems are designed to evolve with emerging threats, ensuring that security measures remain effective over time. By incorporating these systems, organizations can future-proof their cybersecurity strategies, adapting to new challenges as they arise.
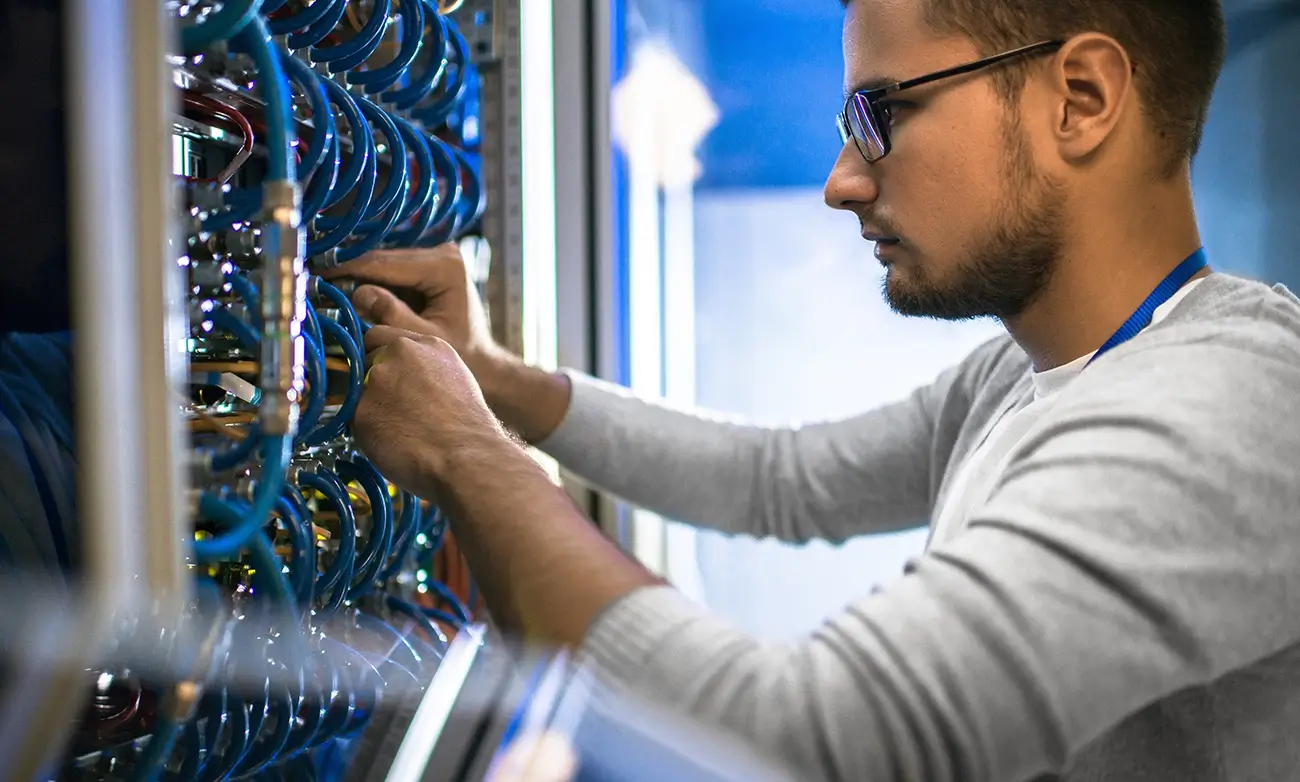
Future of Machine Learning in Cybersecurity
The future of Machine Learning in cybersecurity is bright, with advancements leading to more progressive, automated systems. The combination of ML with technologies like blockchain and IoT promises to boost security capabilities. Organizations need to keep up with these developments, integrating it as a key element in their cybersecurity strategies.
Furthermore, to address increasing industry challenges including IT staff shortages and the incremental amount of data a company collects to detect malicious behaviour that makes it more difficult to detect deceptive and unknown attack patterns and the so-called "needle in the haystack", organizations are turning to predictive analytics and Machine Learning driven network security solutions. In fact, ML-based network monitoring has become an essential tool for securing their networks against cyber threats and the unknown bad.
Predictive Analytics and ML-Driven Solutions
“We, at Exeon, leverage machine learning algorithms and its baselining capabilities for the NDR (Network Detection and Response) platform ExeonTrace. The application of ML ranges from detections for domain generation algorithms to traffic volume analytics, command-and-control channel detections and identifying lateral movement.
In our blog post on the future of network security, we explain the general benefits of using ML and predictive analytics.”
– Andreas Hunkeler, Head of Professional Services

ExeonTrace: A Game-Changer in Cybersecurity
ExeonTrace, a premier Network Detection and Response solution developed by Exeon, harnesses the power of Machine Learning to revolutionize cybersecurity.
By addressing various IT security challenges, ExeonTrace not only assists security teams in their routine tasks but also significantly elevates an organization's cybersecurity posture.

Cutting-Edge with Advanced Threat Detection
Leveraging Machine Learning algorithms ExeonTrace identifies sophisticated cyber threats swiftly. Its capability for rapid network anomaly detection sets it apart, providing a critical edge in the fast-paced realm of cybersecurity. This advanced detection is a testament to the power of machine learning in identifying and reacting to cyber threats in a timely manner.
How ML Algorithms Protect Hospitals From Cyber Attacks
ExeonTrace NDR has helped Solothurner Spitäler AG detect and respond to attacks quickly, securing the network of four hospitals and patient data. Learn about its benefits in a case study with Patrick Käppeli, Network & Security Engineer.
ExeonTrace - The Future of Cybersecurity
Incorporating ExeonTrace into a cybersecurity strategy offers a modern approach to digital threats. Leveraging Machine Learning and AI, ExeonTrace improves threat detection and response, adding sophistication and efficiency to security operations. As cyber threats evolve, ExeonTrace is key for organizations aiming to stay ahead and effectively protect their digital assets.
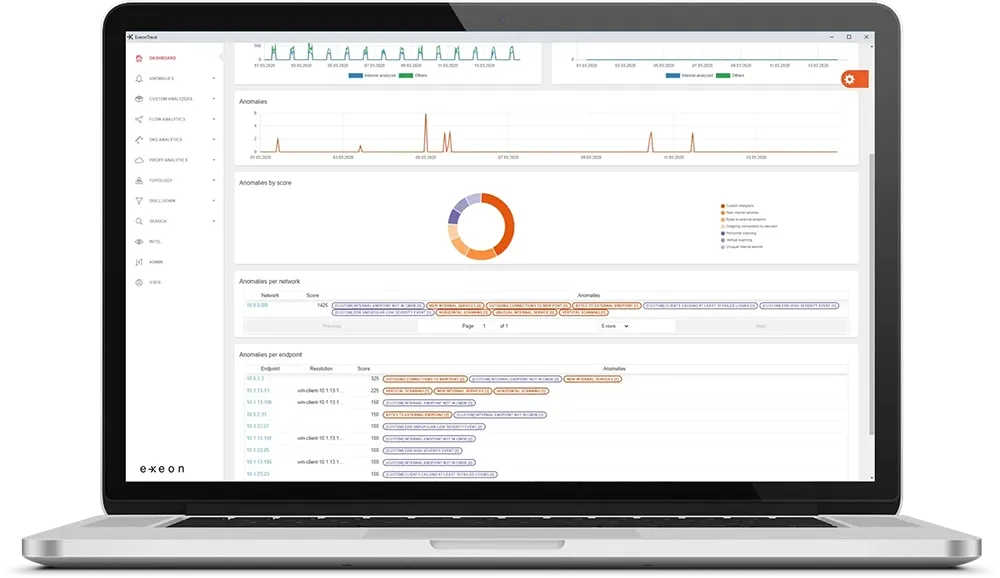
More IT Security Articles From Our Industry Experts
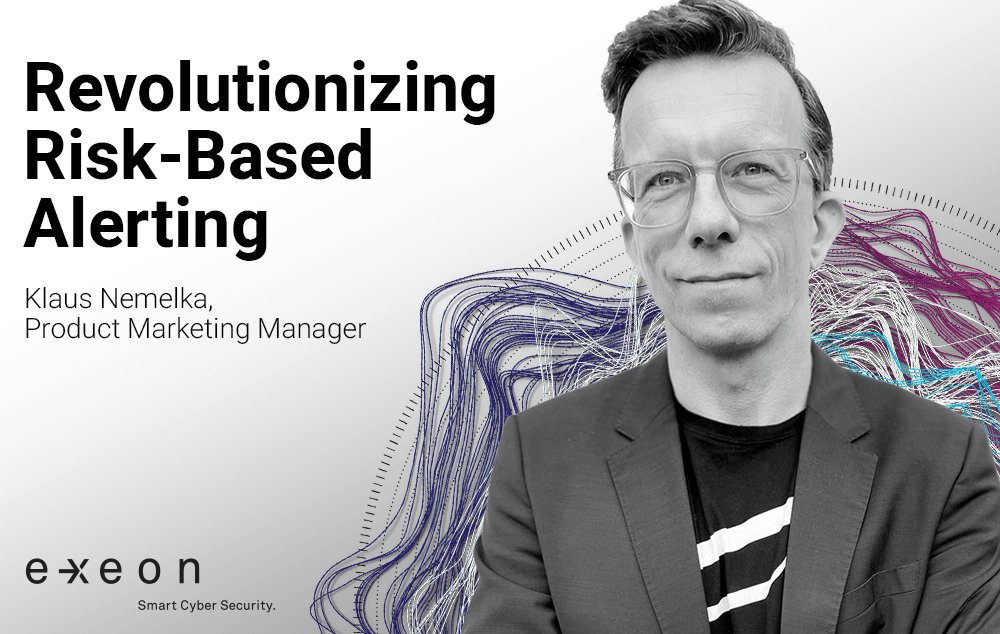
28.11.2023
Enhancing Security Detection: Revolutionizing Risk-Based Alerting
Risk-based alerting (RBA) is a strategy that uses data analysis and prioritization to issue alerts or notifications when potential risks reach certain predefined levels. Find out how to best utilize it for improved security – even against the most advanced attackers.

25.10.2023
How to Catch Data Exfiltration with Machine Learning
Find out how to catch data exfiltration thanks to Machine Learning and why detecting it is crucial to your organization’s IT security, as explained by our Head of Professional Services, Andreas Hunkeler.
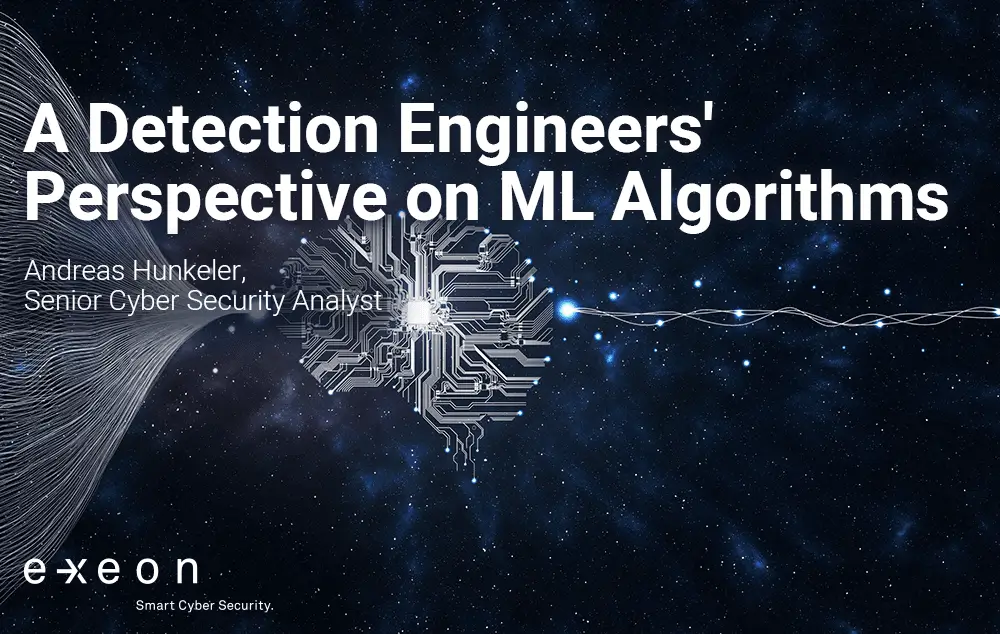
27.06.2023
Machine Learning Algorithms from a Detection Engineers' Perspective
Besides the general discussion about the benefits and challenges of using machine learning algorithms for threat detection, detection engineers face their own benefits and challenges when building detections. In this blog, these aspects are outlined from a detection engineer's perspective and the corresponding questions that come up during use case development.